Demystifying Black Box AI And Its Use Cases
May 16, 2024
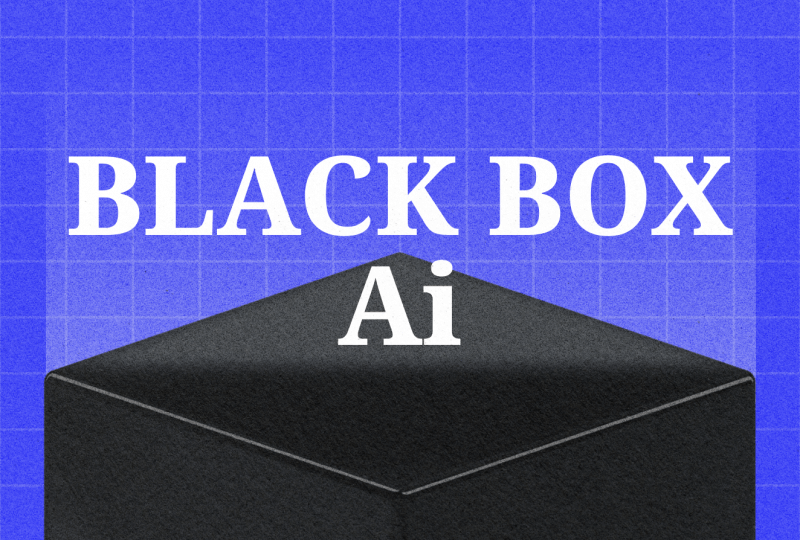
Artificial Intelligence (AI) is revolutionizing the digital realm, with the concept of Black Box AI gaining significant curiosity. This enigmatic term raises questions about its meaning, operation, and implications. Researchers are attempting to integrate black box models with widely accepted white-box models to overcome their hidden nature and gain legitimacy in machine intelligence systems despite their high accuracy.
This article explores the concept of blackbox AI, its challenges and restrictions, as well as spheres where a black box model can be used.
Key Takeaways:
- Blackbox AI model refers to software that employs complex algorithms that cannot be simplified into comprehensible rules and applications.
- AI black boxes are composed of three primary components: machine learning algorithms, computational power, and data.
- Black Box AI can be used in numerous spheres, including finance, healthcare, and vehicles.
- The key challenges of black-box AI are lack of transparency, liability, biases, and ethical concerns.
Clarifying Black Box AI
Black-box machine intelligence models are AI systems where the algorithms’ internal workings are hidden or unknown. It refers to software that uses data that is not accessible to the data subject or uses complex algorithms that cannot be reduced to comprehensible rules and applications.
These systems lack transparency and may not allow for intuitive explanations. Deep learning algorithms, which focus on training deep neural networks, are often used for representations in tasks like image and speech recognition, natural language processing, and reinforcement learning.
Black box AI can be caused by proprietary IT and deep learning models, which create thousands of non-linear relationships between inputs and outputs. This complexity makes it difficult for humans to explain which features or interactions led to a specific output. Black box AI can be useful in complex scenarios where human interpretation may be limited.
For example, in the financial sector, it is commonly used to detect fraud by rapidly analyzing massive amounts of data to identify patterns and anomalies.
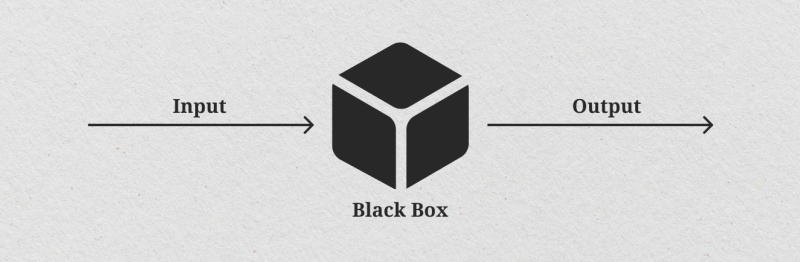
Despite their effectiveness, black-box machine-learning algorithms and models are often considered dangerous due to their potential to be manipulated. For instance, if a machine-learning model used in health care diagnoses your health, you might want to know how it arrived at its decision.
These models, while accurate and effective in predicting sectors like finance, healthcare, and the military, are not widely accepted due to their lack of transparency and interoperability.
How It Works
Artificial neural networks, inspired by biological neural networks, are machine learning models with interconnected nodes organized into layers.
AI black boxes consist of three main components: machine learning algorithms, computational power, and data. Deep learning algorithms enable AI to learn from data, identify patterns, and make decisions based on them. These systems require significant computational power to process large amounts of data.
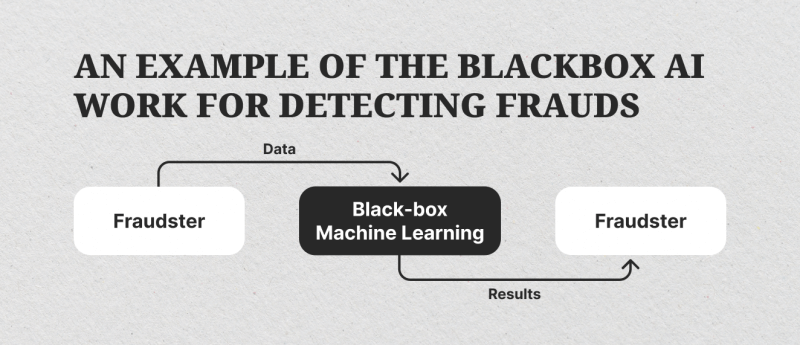
AI black boxes can be trained by fine-tuning algorithms and customizing data. During training, systems are exposed to relevant datasets and example queries to optimize performance. Once trained, black box AI can make independent decisions based on learned algorithms and patterns. However, transparency about decision-making processes remains a concern.
Fast Fact:
AI facial recognition software in law enforcement is found to be statistically biased against people of color, though it should not have a concept of race or skin color to reduce the likelihood of racist decisions.
Black Box AI Use Cases
Black Box AI excels in complex tasks and accurate predictions, with numerous applications across various fields. Here are some of the most common spheres where Blackbox AI can be used.
Finance
Financial institutions have increasingly used AI to reduce risks and optimize efficiency in areas like Anti-Money Laundering, Counter-Terrorism Financing, risk management, and market abuse. Artificial Intelligence is also being used by traders to gather information, such as trading bots in cryptocurrencies, to provide precise trading instructions.
However, the use of AI models in highly regulated, opaque, and uncontrollable sectors requires interpretability and explainability. This is crucial for societal trust and openness in the financial sector. Efforts have been made to increase transparency in AI decision-making capabilities, such as predicting abnormal expenses and using Generalised Linear Models to determine insurance prices. XAI is also being used in other sectors as the importance of explainability grows. Another issue is that traders need to be aware of market movements and currency news, which AI Black-boxes cannot provide.
Healthcare
Black-box technologies are increasingly being used in the healthcare industry to access vast amounts of data, enabling systems to acquire complex knowledge that humans may not understand. For instance, X-rays can identify broken bones in hands, allowing doctors to analyze data and provide visuals of the problem. This technology has gained popularity in healthcare due to its ability to access vast amounts of data.
Black Box AI is utilized in diagnostics, drug discovery, and personalized treatment plans, analyzing medical imaging data to detect disease signs that human doctors may miss.
However, user acceptability remains a concern. To address this, an explanatory component was introduced, providing appropriate reasoning for predictions based on the patient’s medical history. This has helped establish confidence and increase patient safety in the healthcare industry.
Business
Black Box AI is a significant tool for business professionals, providing insights into complex data and predicting market trends. However, due to unclear reasoning, it can be challenging for professionals to trust their decisions. They must balance AI’s use with their own expertise and judgment, recognizing it as a tool rather than a replacement for human decision-making.
Vehicles
Automated transportation has become a reality with the development of AI, which can eliminate unsafe driving behaviors. However, dependable and secure autonomous cars still lack the cognitive skills of humans. A 2016 incident where a Tesla on autopilot failed to cross an 18-wheeler truck on a highway highlights the need for comprehensible self-driving systems.
Recent studies have attempted to build trust in self-driving vehicles by demonstrating that passengers feel more comfortable when the car abruptly changes lanes. Black Box AI is crucial for autonomous vehicle operation, enabling real-time processing of sensor data and making driving decisions.
Legal System
AI is increasingly being relied upon in law enforcement, investigations, and punishments. Black box technologies interpret DNA mismatches and perform facial recognition and risk assessments for recidivism. These complex and secretive technologies influence how judges, juries, and policymakers think about evidence in court cases.
However, the reliance on these technologies is problematic as they can be misunderstood and even incorrect. AI explanations are not always accurate, as many explainable methods disagree with each other, though some advocates argue that some mistakes are worth tolerating due to the accuracy provided in other cases.
Black Box AI Risks And Concerns
Black Box AI is transforming our lives through virtual assistants and many impressive capabilities, but it also presents numerous challenges and risks that require immediate attention.
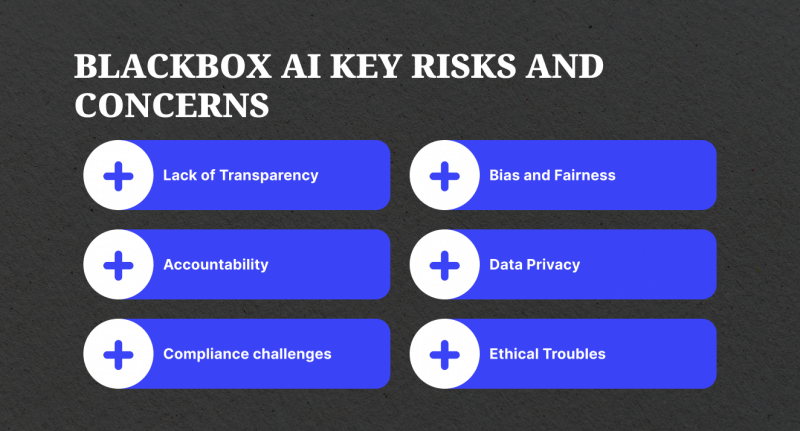
Lack of Transparency
Black box AI lacks transparency, making it difficult for users to understand the reasoning behind specific AI-driven decisions, particularly in critical fields like finance and medical care, which makes it difficult to trust their outcomes.
Bias and Fairness
Black box AI models, trained on extensive amounts of data, can potentially perpetuate biases and discrimination due to their hidden decision-making process, making it challenging to identify and rectify such biases.
When trained on biased data, AI systems can unintentionally reinforce these biases, leading to unfair practices and negative consequences for marginalized or underrepresented groups. Black-box AI makes it difficult to detect this behavior.
Accountability
The opaque nature of a Black Box AI system makes it difficult to hold anyone accountable for incorrect decisions or predictions.
AI models can be difficult to blame or identify the root cause of critical errors or harm when their decision process is concealed.
The absence of accountability in AI systems can lead to legal complications, regulatory challenges, reputation damage, and financial losses.
Data Privacy
Black Box AI systems often require significant amounts of data for training, raising concerns about data collection, usage, and storage, potentially posing privacy risks.
Black box systems can also be vulnerable to attacks or exploitation without understanding their internal operations.
Compliance Сhallenges
Regulatory bodies and governments are scrutinizing AI apps, highlighting the potential challenges of black-box AI in ensuring transparency and liability in compliance with regulations.
The lack of clear accountability or explanation of AI outcomes makes it challenging to meet regulatory or compliance requirements.
Ethical Troubles
The black box problem in AI raises ethical worries about its decision-making procedure. Understanding how AI algorithms make decisions is crucial for fairness and avoiding discrimination. Researchers are exploring ways to improve the transparency and interpretability of AI systems, such as developing “explainable AI” (XAI) and using machine learning approaches to help humans understand and identify biases.
The possible ethical considerations of black box AI in criminal justice and employment are substantial, and cooperation between researchers, practitioners, and policymakers is essential for developing guidelines and regulations for responsible and ethical use of these powerful systems.
Black Box and White Box: How They Differ
Black Box AI and White Box AI are subfields of AI, but they differ in transparency, interpretability, and complexity.
Blackbox AI is a system that lacks transparency, with its inner workings hidden from the user or operator. This type of AI is often used in complex situations with vast data, such as image recognition tools, voice recognition software, and self-driving cars. These technologies can be challenging to understand, but their capabilities are impressive.
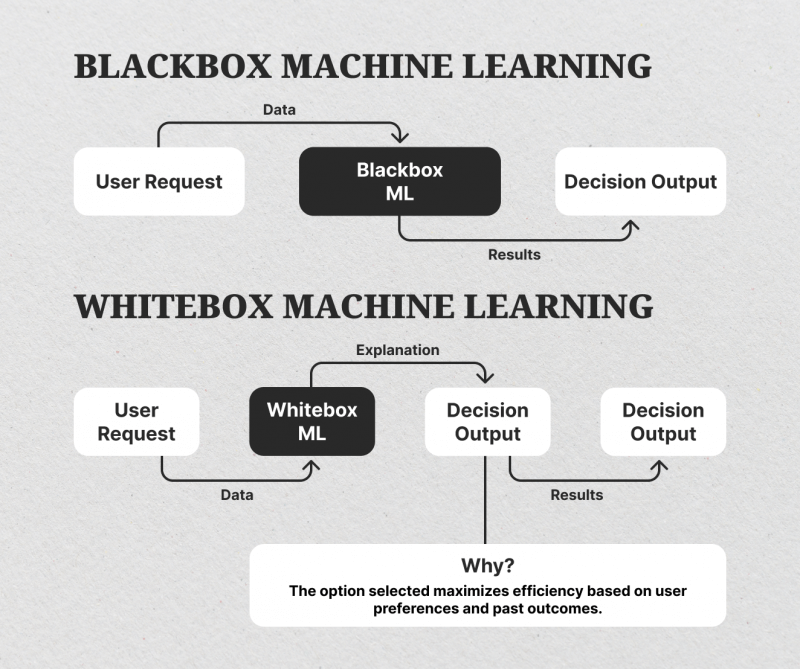
Unlike black box, white box AI, also known as ‘Interpretable’ or ‘Explainable’ AI, is transparent about its conclusions, allowing data scientists to understand algorithms and decision-making factors. As people become suspicious of black-box AI, white-box models have gained popularity.
They are practical for businesses, helping them improve workflows and identify potential issues. White box AI’s linear insights are less radical and may not provide game-changing ideas. Despite their lack of technical sophistication, their transparency offers a higher level of reliability and trust for end users.
White-box AI is used in situations where clarity and comprehensibility are crucial. Examples of white-box AI include decision trees, rule-based frameworks, and linear regression models. These systems aim to be more transparent, allowing users to understand the reasoning behind their decisions. White-box AI’s openness allows for easier examination and modification of the underlying algorithms, ensuring fair and ethical decisions.
When choosing between black-box AI and white-box AI, companies can adopt a hybrid approach that combines the strengths of both methods. White-box techniques can reveal the inner workings of black-box AI systems, while black-box strategies can enhance white-box AI systems’ capabilities.
This balance between effectiveness and transparency is crucial. Regardless of the strategy, companies should prioritize transparency, accountability, and ethical decision-making to foster trust and unlock AI’s full potential for innovation and growth.
Final Thoughts
AI has revolutionized many spheres, such as the finance sector, healthcare, education, and more. Black box AI models are being utilized in various fields, including investing, healthcare, banking, and engineering, as they develop alongside machine learning capabilities. These models are becoming more opaque, relying on results without understanding how they are produced, resulting in increased complexity in their processes.
Blackbox AI has expanded AI possibilities but presents challenges in transparency, fairness, and accountability, as well as ethical dilemmas. As AI technology evolves, developers, organizations, and regulators must address these challenges and strive for transparency and fairness in AI systems to maximize benefits while minimizing risks.
FAQs:
How does white-box AI and black-box AI differ?
White Box AI promotes transparency in decision-making processes, while Black Box AI conceals internal workings, making its decision rationale less transparent.
Where is white box AI applied?
White Box AI is gaining traction in crucial sectors like the medical field and finance, where trust and interpretability are crucial for decision-making and compliance.
What can Blackbox AI do?
The black box is a complex system that offers superior prediction accuracy in computer vision and natural language processing due to its ability to identify intricate patterns in data.
How accurate is black box AI?
Despite their complicatedness and lack of transparency, Black box AI algorithm results are known for their highly accurate outputs.